Dynamic Pricing of Seat Assignments
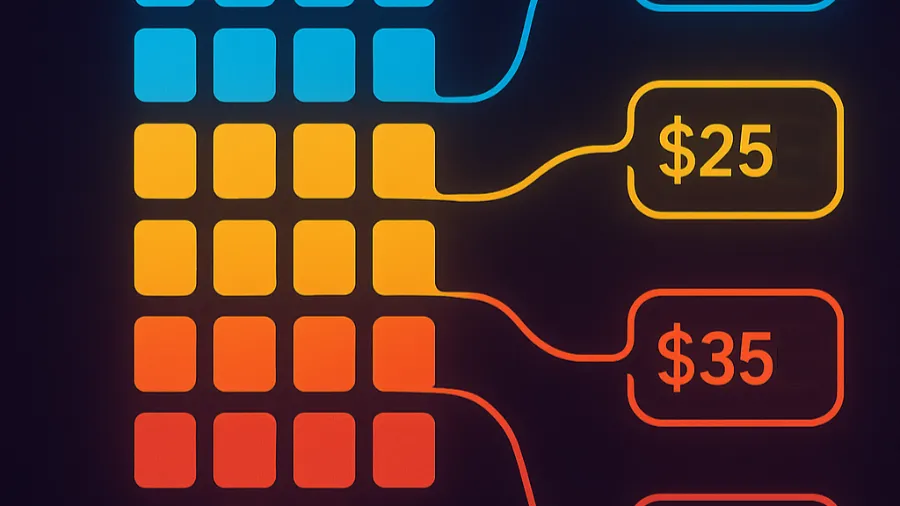
There has been much industry discussion about continuous pricing, perhaps the most important subset of the overall trend toward dynamic offers. However, continuous pricing is as difficult as it is valuable. As we pointed out recently, it requires a lot of strategic thought and a multi-faceted implementation approach across channels. For many airlines, it is not the best place to start their journey toward dynamic offers.
Where is the best place to start? In our view, the lowest hanging fruit lies with dynamic pricing of seat assignments. In this piece, we will explain how we came to that conclusion, how to bring it to life, and what results an airline can expect at the end of the rainbow.
Why Start with Dynamic Pricing of Seat Assignments?
While every airline’s business model varies to some degree, the top a la carte ancillaries (excluding the sale of loyalty program miles) for most are checked baggage, fees for fare flexibility, and paid seat assignments. It makes sense, therefore, that we would find the lowest hanging fruit among the top three. Of these, paid seat assignments stand out in that they are both (a) largely discretionary items and (b) consumed most often at the original point of sale. The demand for the other two are more inelastic and determined much later in the travel journey.
Paid seat assignments, like the underlying travel from point A to point B, have a wide range of value across various customer segments according to their needs and preferences. A person traveling alone on a 30-minute flight has a different willingness to pay from a party of two traveling together on their wedding anniversary, from a businessperson who is rushing to complete a presentation for the big meeting scheduled an hour after their arrival. Airlines have long employed customer segmentation and used data-driven systems to optimise pricing. Dynamically pricing seat assignments is a natural extension.
These are all good reasons to start with paid seat assignments, our favourite is that seat assignments are done in standalone, self-contained flows which do not intersect with or complicate flight shopping. This gives an airline wide latitude to revamp its seat assignment experience across all direct and NDC-enabled channels without touching core aspects of shopping and fulfilment. Implementations are therefore lighter and changes nimbler.
Finally, there is less worry about external factors with seat maps than there are with flight shopping displays. With continuous pricing, an airline must worry about how its continuous prices compare to its static pricing in EDIFACT channels. With paid seat assignments, there are typically no prices in EDIFACT seat maps to which to compare. With continuous pricing, an airline must worry about competitive pricing levels as their continuous prices will be displayed side-by-side with competitors’ prices. This necessitates robust ingestion of competitive pricing information to inform continuous offers. Seat maps, on the other hand, are displayed to a captive audience and real time ingestion of competitive prices is less relevant though, of course, having competitive prices in general remains important.
How Can an Airline Bring Dynamic Pricing of Seat Assignments to Life?
The key component required to dynamically price seat assignments is a machine learning-based algorithm which learns from the offers which are made, the customers which receive them, the contextual circumstances which surround them, and the resulting successful or failed sales. This type of closed loop reinforcement learning is critical to gradually improving offers over time.
If an airline already has a merchandising tool which sits behind its direct or NDC-powered channels, the merchandising tool may already have a native machine learning algorithm. If it does not, an algorithm can often be bolted onto the existing merchandising tool. In this case, the merchandising tool becomes the central storehouse of all static pricing, and the machine learning algorithm can interject dynamic prices when its system is called.
Once the machine learning algorithm is in place, some initial set up is typically required. For example, the airline may already have an idea of which customer and/or contextual characteristics can be used to differentiate pricing between situations in which customers may have higher or lower willingness to pay for certain seat assignments. It helps to give the algorithm a head start by configuring these in the system. The airline may also want to set some initial or permanent guardrails on the range of pricing that can be offered in various circumstances, such as setting pricing floors to prevent customers from gaming the system with repeated requests.
With the system installed and initial configuration complete, the airline can begin to filter a small amount of volume to the system. It is important to have flow controls set up within direct and NDC-powered channels to ensure that the system’s launch is not all or nothing. The airline should feed a small fraction of volume to the system initially, and its commercial, operational, and revenue accounting analysts should watch its behaviour closely to validate the system is functioning as expected.
Once the system is validated with limited volume in production, the airline may proceed with more robust A/B testing. This may begin with roughly half of the volume being fed into the new system, with commercial analysts watching daily or intra-day data to ensure that the new system is providing improved revenue results and higher conversion. As the airline becomes more comfortable with the system’s performance, it can begin to favour the dynamic offers over its static offers.
What Results Can an Airline Achieve from Dynamic Pricing of Seat Assignments?
The downfall of static pricing is that for some customers it is too high, resulting in lost sales. For other customers, it is much lower than they would otherwise be willing to pay, resulting in money left on the table by the airline. Segmented, dynamic pricing powered by machine learning can result in more sales at higher average prices. A recent case study published by PROS and airBaltic, revealed the total uplift of paid seats revenue was 6 percent, much higher than the 2-3 percent they initially expected.[1]
A secondary benefit is that it makes the revenue management and/or merchandising analyst team more productive with fewer errors. Static pricing is time consuming to create and maintain, and any mistakes in how it is configured can cost the airline a large sum of money. As the airline transitions to depending more upon machine learning, the workload on the analyst team is gradually reduced, as are the number of errors.
Finally, an indirect benefit is that machine learning can put a more accurate price on the seat assignments which are provided for free either as part of fare bundles or in connection with the traveller’s loyalty status. This can result in smarter trade-offs between what is provided at a price versus for free and can even lead to more optimal prices of the fare bundles themselves.
Conclusion
Implementing dynamic pricing for seat assignments provides a sensible starting point for airlines exploring flexible offer models. This method enables early revenue wins from pricing adjustments that reflect customer needs, driven by innovative technology, without the need to reengineer the core booking process. Early gains in revenue and efficiency underscore its promise, and it paves the way for enhancing the value of free services and bundles, equipping airlines to wisely navigate their loyalty program proposition and diversifying product lines.
[1] “How airBaltic is Driving Seat Revenue with AI-Powered Dynamic Ancillary Pricing” by PROS. Retrieved from https://pros.com/learn/case-studies-testimonials/how-airbaltic-driving-seat-revenue-with-ai-powered-dynamic-ancillary-pricing